Hume Center and SRC, Inc. Demonstrate Real-World Applications of Radio Frequency Machine Learning Technologies
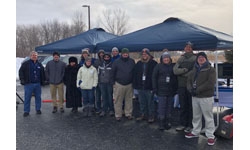
On December 19, 2019, the Ted and Karyn Hume Center for National Security and Technology at Virginia Tech and SRC, Inc. showcased the results of their year-long collaborative effort in developing Radio Frequency Machine Learning (RFML) techniques for performing wireless signal identification on real-world signals of interest. During this “balmy” Syracuse December day (with wind chills reaching -3° Fahrenheit), the team set up their hardware and software solutions outside and used real-world signal sources to test the algorithms developed over the course of the program, achieving extremely high accuracy in both signal detection and identification tasks.
The primary focus of this collaboration was to compare and contrast two of the more common architectures for deep learning, namely convolutional neural networks and recurrent neural networks, applied to this unique RFML application. The trade-offs between each approach were identified through analysis, as well as this real-world demonstration, to better understand the trade space of each architecture given the particular applications and environments of interest.
The Hume Center team consisted of the following members of the Electronic Systems Lab: PI Dr. William “Chris” Headley, Co-PIs Dr. Joseph Gaeddert and Dr. Alan Michaels, PMs Deanna Clark and Alexandra Poetter, Faculty Researchers Lauren Wong and Bill Clark, and Graduate Research Assistant Megan Moore.
Radio Frequency Machine Learning (RFML) is the unique application of state-of-the-art deep learning techniques to solve wireless communication problems using RF data for training, validation, and evaluation. The Electronic Systems Lab has garnered more than $5M in research funding, generated 10 publications, and produced multiple graduate student theses in recent years in this exciting research area.